Anyone who has ever been to Las Vegas or Los Angeles knows firsthand the meaning of urban sprawl: an overdose of concrete and asphalt, wasteful use of land, meager open space–all because of unplanned, out-of-control growth. The specter of L.A. clones has terrified many a city planner throughout high-growth areas in the West and South, but preventing such a nightmarish outcome is no easy task. City planners face a Pandora’s box of competing interests, countless options, and elected officials who are untrained in city planning but nevertheless have decision-making power.
An alliance of BYU professors is hoping to help with a novel approach to land-use planning that uses computers to wed city streets and genetic theory. The idea is to apply to city planning the theory of evolution: refine species by throwing out bad traits and keeping good ones.
The process is called optimization, and scientists increasingly are using it to help them design complex items ranging from jet engines to bicycle brakes. “It’s a way to use computers to search through all the different possibilities and get to the best one faster rather than just by trial and error,” says Richard J. Balling, professor of civil engineering and one of three principal investigators on the project. Balling has worked on optimizing the design of skyscrapers and micromachines used in eye surgery, but transferring this relatively new technology to land-use planning is a first for him and a first for the field. And it’s a first that has the potential to dramatically reshape city planning, says Richard H. Jackson, chair of BYU’s Geography Department and another of the project’s principal investigators.
The type of optimization the BYU researchers are using is called a genetic algorithm. An algorithm is simply a computational procedure, and a “genetic” algorithm manipulates the computations with genetic principles.The technique goes something like this: Take one possible master plan for a city with its dozens of zones and traffic corridors, each a “gene,” and call it the “mom” chromosome. Take a second plan, the “dad” chromosome, mate it with the mom chromosome, and you get offspring that just might include a better master plan than either of the parents. Manipulate this “breeding” process with mutation and cloning, weed out inadequate plans, run it on a computer for dozens of “generations,” and you’ve got the ultimate in computer-aided asexual “reproduction”–and you might very well come up with much better land-use plans than a mere human could ever devise. It’s a simplistic reproductive scheme, to be sure, but using the genetic algorithm gives planners a higher probability of finding the best plans because it works from previously good plans to create better plans.
Balling, Jackson, and fellow researcher Lawrence C. Walters, director of the Marriott School’s Romney Institute of Public Management, have just finished a four-year project that applied this technique to the city of Provo. Funded by the National Science Foundation, the project has involved about 10 graduate students and produced several papers accepted for publication, including one that appeared in the June 1999 Journal of Urban Planning and Development.
Planning for Provo
The population of Utah in general and the Provo-Orem area in particular have been growing at unprecedented rates since an economic boom began in the late 1980s. Some 80 percent of Utah’s 1.8 million people live along the Wasatch Front, a narrow strip of land from Provo to Ogden, hemmed in on the east by mountains and on the west by Utah Lake and the Great Salt Lake. Those geographic limits make it especially important that the area plan for the tremendous growth expected during the next two decades.
Though some of the growth is the result of an influx of people from other states, most of it is caused by a high birthrate. Natural birth alone will shoot Provo’s population of about 108,000 to 192,000 within 20 years if current growth rates continue, says Balling. The city’s existing streets and zoning plan won’t accommodate that population, so streets will have to be widened and zones changed to allow for higher-density housing. But which streets and which zones? The city has 130 zones, each with 10 possible land uses–residential (very low, low, medium, and high density), central business district, shopping center, general commercial, light industrial, and heavy industrial. The 25 main traffic corridors also have 10 possible uses each, from two-lane collector to six-lane arterial. With so many options the number of possible city plans is virtually endless–10155 to be exact. Searching through a reasonable portion of that incomprehensible number with human power alone would be more than a lifelong task.
The human limitations on such a search point to one of the major problems with city planning, says Balling: planners search too few plans. “It’s very subjective. Instead of searching through 2 million possible plans, as we’ve done, they might come up with only a handful of plans, which is a very limited choice. The computer technology and algorithms are to the point now where they can search over millions of plans to come up with better ideas.”
Applying computer power to this problem was the goal of the BYU researchers. But the professors decided that before they shut themselves up in the computer lab, they needed to understand the human side of things. Land use is, after all, a people-centered concern–and a very thorny one at that, especially in a conservative state where property rights are held sacred. So they started poking around the political process and meeting local decision makers who have responsibility over planning.
“We wanted to find out who the players are here in Utah and what they’re doing. We interfaced a lot with local and state planners and politicians to find out what’s going on,” says Balling.
The group also developed a survey to find out more about public sentiment on the topic of growth. “We needed to know what people want,” says Balling. Larry Walters took responsibility for this phase, developing a survey that went out to 10,000 households in five Wasatch Front counties (see “Exploring Public Perspectives of Growth,” below). The survey results helped the researchers choose three objectives to use in evaluating city plans: minimize traffic congestion, minimize change, and minimize cost. To create models that would help them judge the quality of the plans, they pulled together technical experts on everything from traffic congestion to water use. For example, BYU alum John Taber of Tabermatics, a transportation consulting firm in Park City, Utah, was key in developing a computer model to calculate projected travel time for each hypothetical plan.
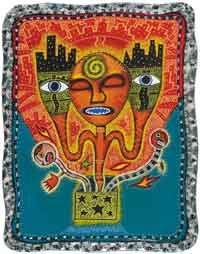
City planners face a Pandora’s box of competing interests, countless options, and elected officials who are untrained in city planning yet have power over decisions.
The Genetic Algorithm Tool
With the objectives and models in place, the group developed the genetic algorithm. They weren’t looking for one single stupendous plan, because no one plan could possibly be ideal in all respects–optimizing one objective is almost always achieved at the expense of another objective. A plan with the best possible traffic flow, for example, could not also be the most economical plan. Instead, they were looking for a collection of plans where each plan is optimal for a given objective or mix of objectives. This final set of plans is called a “Pareto-optimal” set, named for the 19th-century Italian economist Vilfredo Pareto. A plan became a member of the Pareto set if no other plan existed that was better in every objective. Using a Pareto set, planners can choose which objectives they want to weight most heavily and then find the Pareto plan that matches their priorities.
“The beauty of the Pareto set is that it is independent of the relative importance of the objectives,” Balling wrote in a journal article about the project. “For a given combination of weighting factors between objectives, the optimum plan will be one member of the Pareto set. For another combination of weighting factors, the optimum plan will be another member of the Pareto set.”
So, how did Balling et al. create their Pareto set? Because it’s impossible to search over the entire universe of 10155 plans, they started by taking the current zoning plan for Provo and randomly computer-generating variations until they had 100 feasible plans. A plan was deemed feasible if its zoning provided enough housing for the projected 192,000 Provo population. The group had to generate nearly 2 million plans to glean 100 feasible plans for the starting generation. Then they applied various reproduction principles to imitate the genetic process. Each of the original 100 plans was assigned a fitness ranking depending on a mathematical measure of Pareto optimality. The plans with highest fitness were weighted more heavily so that it was more likely they would propagate. To create the second generation, the computer randomly paired up plans from the starting generation.
“You could think of it as each plan getting space. The plans with the highest fitness have more space on the roulette wheel. You spin the wheel, and wherever the marble lands, that’s the plan you pick as the father. You spin it again and get a plan for the mother. Plans with the highest fitness will be mother and father more often, so it’s more likely that the most fit plans will become parents–but others have a chance,” says Balling.
It was important to allow the less-fit plans to become “parents” as well, because though they may not have been great plans overall, they could have had a few good features that would benefit subsequent generations. “You need this genetic diversity,” he says.
Mating the plans was a relatively simple process. Each plan for Provo can be represented by a chromosome with 155 “genes”–one for each of the various zones and traffic corridors in the city. The value of each gene indicates the use of the corresponding zone or corridor. To “mate” the two selected plans, then, the researchers randomly generated a number between 1 and 155, a different number for each pair. At that gene, they snipped each parent chromosome and swapped the tail ends, yielding two offspring that had many of the same characteristics as the parents, but also some variations. Immediately they checked to see if the progeny could handle the housing needs of 192,000 people. Any plan that failed the housing test was discarded. Then they spun the wheel again, picked two more parents, and created more descendants. The algorithm also employed such biological devices as mutation and cloning. Mutation, used sparingly, injects the opportunity for something new and unexpected. “The starting population might not include the best traits, so mutation ensures diversity,” says Balling. And cloning guarantees that the best plans from one generation aren’t lost in the genetic shuffle. The 10 most fit plans in each generation were identified and copied over to the next generation.
A generation was considered complete when it yielded 100 feasible plans, which were then assigned fitness rankings and run through the process to create yet another generation.
Deciding exactly how to fashion the genetic algorithm was something of an art, says Balling. “There’s no right way or wrong way. How many generations, how many in each population, the probability of mutation–all of these things are parameters that we set.”
He and his colleagues decided to run 100 generations of 100 feasible plans, yielding a total of 10,000 feasible plans, which were then pared down to a Pareto set of 320 plans. As anticipated, the plans in the last generations were significant improvements over the starting plans in terms of the three objectives. “The average travel time, cost, and amount of change improved significantly from the starting plans and even from the mid-generation plans. If we had stopped at 50 generations, we wouldn’t have found these plans,” says Balling.
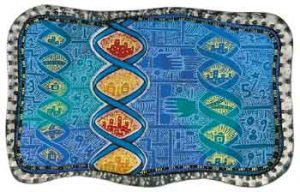
The Genetic algorithm gives planners a higher probability of finding the best plans because it works from good plans to create better plans.
The Real-World Test
Once the Pareto set was built, the problem arose of how to choose a single plan from among more than 300. The researchers felt that such a large number of plans might overwhelm decision makers, so they added yet another component to the project–a computer tool that enables planners to look at a city map on a computer screen and lay Pareto plans on top of it one at a time. Slider bars on the program let planners “morph” the map according to the objectives they want to emphasize. If they want to see the best plan for keeping travel time low, for example, they give maximum weight to travel time, and then they monitor what happens to their other objectives.
“When this tool is done, you’ll be able to play around with these slider bars, and the map of the city will change right in front of your eyes,” says Balling. “A planner could observe trends. For example, if I want to minimize costs, what will happen in the northeast part of the city? If I trade off costs with travel time, then what happens to that part of the city?”
The tool, nearing completion, is called the “Pareto set scanner.” Planners will be able to sit in a room, use the scanner, and see what happens to the city if they emphasize one objective over another. “They can hammer on the table and argue with each other about what’s most important, but we’ve kept their focus on the best plans. They’re not looking at any bad plans,” says Balling.
Balling emphasizes that the group’s effort is research–an academic, futuristic approach to planning that may or may not be useful in real life. “Our role as university researchers is mostly to come up with cutting-edge ideas. The commercialization or actual implementation of those ideas is left to planners and consultants.”
Real-life planners have had a chance to review the research at several urban planning conferences, including the annual Transportation Review Board conference held in Washington, D.C., last January. So far they are reacting with surprise and curiosity. “It’s kind of fun when we present this,” says Balling. “The jaws just drop. We start talking about chromosomes and mutation and reproduction, and everybody is just amazed.”
The National Science Foundation, for one, is interested. The foundation has awarded the BYU team a second grant that will allow the researchers to extend their project to the entire Wasatch Front. Joining the group for the second phase is new civil engineering faculty member Mitsuru Saito.
Team member Richard Jackson is as qualified as anyone to assess the genetic algorithm’s pragmatic value, as he was on the Orem City Council for eight years, has served on Orem’s Planning Commission, and is a member of the American Institute of Certified Planners. He believes it could be extremely useful to those who apply it well.
“This tool is the only thing of its kind in the planning field, and it has the potential of being perhaps the most valuable tool that city councils have in making their decisions,” Jackson says. “That is, if they’re willing to put the data into it and then make the hard decisions, which means deciding what their priorities are.”